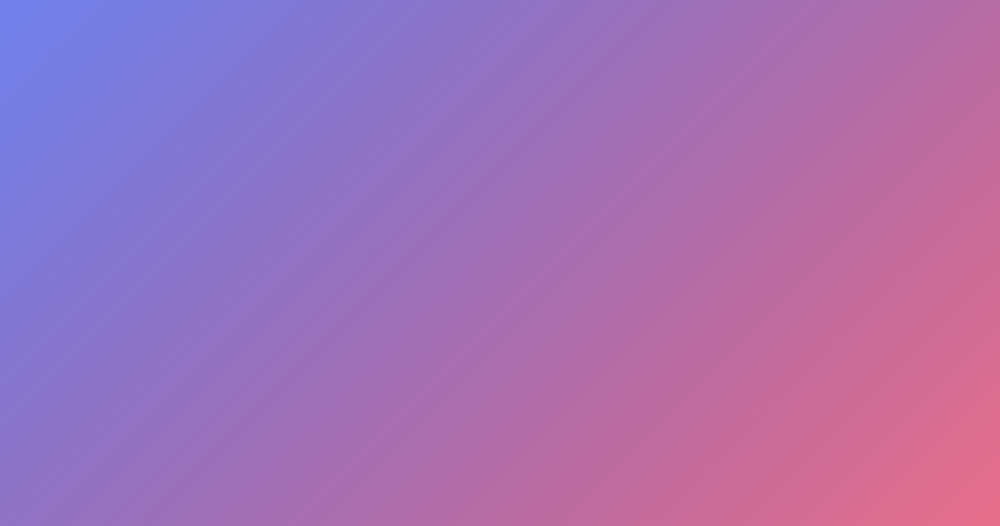
13
四月Data Vizualization Tools: Visualizing Data Distribution
Data Insights
Histograms are a type of graphical representation that displays the distribution of numerical data. They are used to chart the frequency of data points in a particular range or bin, allowing us to understand the shape of the distribution, locate patterns, and make informed decisions based on the data.
To create a distribution graph, we need to divide the range of the data into equal intervals, also known as bins. Each bin represents a range of values, and the height of the bar in the graph is proportional to the number of data points that fall within each bin. The x-axis represents the bins, and the y-axis represents the frequency or count of data points in each bin.
One of the key features of data visualizations is that they can show the distribution of the data graphically. If the data is normally distributed, the chart will be symmetrical and bell-shaped. A skewed distribution, on the other hand, will have a distribution with bars that are asymmetrical.
Data Insights can be used in various fields such as social sciences to understand the distribution of data. For example, Lean Manufacturing consultant in finance, a histogram can be used to show the distribution of stock prices or trading volumes. In physics, data insights can be used to understand the distribution of a machine's performance or the stresses on a material.
However, histograms have their limitations. One major limitation is that they can be inaccurate if not used properly. For instance, if the bins are too narrow or too wide, it can change the shape of the distribution. Therefore, it is essential to choose the correct bin size based on the data.
In addition, frequency charts can be modified to suit specific needs. For example, a cumulative distribution graph shows the cumulative count of data points within each bin, while a percent histogram shows the proportion of data points in each bin. These modified histograms can provide more meaningful insights into the data.
To avoid misleading conclusions and to get the most out of frequency charts, it is essential to follow some best practices. These include choosing the correct bin size, ensuring that the data is representative of the population, and using multiple data interpretation techniques to cross-verify the results.
In conclusion, data visualizations are a powerful tool for visualizing data distribution. They can be used to understand the shape of the data, locate patterns, and make informed decisions. However, it is essential to use them judiciously and follow the best practices to avoid any potential pitfalls. By mastering the art of creating informative and accurate frequency charts, we can gain valuable insights into the data and make better decisions.
Reviews